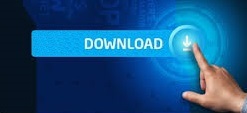

Cartographic generalization simplifies the map content while preserving as much as possible the initial characteristics and spatial relations of the map. We believe that targeting the revealed challenges using the proposed solutions will significantly raise the automation level for producing city wayfinding maps, thus, having a real, measurable impact on production time and costs.Ĭartographic generalization is a process similar to text summarization that transforms a map when scale is reduced. The aim of the paper is to explore those techniques and to recommend future practical studies for each of the identified five challenges in map production. We focus on deep learning for which we provide several examples of techniques from other application domains that might have a potential in map label placement. These techniques are based on quantified cartographic rules or on machine learning. We evaluate these challenges in an open source map labeling tool (QGIS), provide results from a preliminary study, and discuss if there are other techniques that could be applicable to solving these challenges.
CARTOGRAPHICA II MANUAL
In this paper, we identify five cartographic labeling situations that present challenges by causing intensive manual work in map production of city wayfinding maps, e.g., label placement in high density areas, utilizing true label geometries in automated methods, and creating a good relationship between text labels and icons.
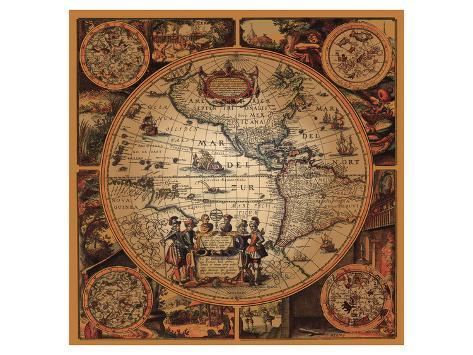
Map label placement is an important task in map production, which needs to be automated since it is tedious and requires a significant amount of manual work. Moreover, the algorithm gained superlinear speedup compared to the previous studies that applied this hybrid algorithm. Our results based on real-world datasets confirm the usability and scalability of the algorithm and illustrate its excellent performance. The optimization component of the proposed algorithm is designed based on the hybrid of discrete differential evolution and genetic algorithms. Parallel computing is then utilized to handle each decomposed problem simultaneously on a separate central processing unit (CPU) to speed up the process of label placement. To address the mentioned challenges, this paper proposes a novel parallel algorithm with the concept of map segmentation which decomposes the problem of multiple geographical feature label placement (MGFLP) to achieve a more intuitive solution. Additionally, in large-scale solutions, the algorithm possibly gets trapped in local minima, which imposes significant challenges in automatic label placement. The complexity of such a problem is compounded as the number of features for labeling increases, causing the execution time of the algorithms to grow exponentially. Multiple geographical feature label placement (MGFLP) is an NP-hard problem that can negatively influence label position accuracy and the computational time of the algorithm. Through an application of the proposed model tested on volunteered geographic (VGI) data and the creation of sample parameter settings, the article illustrates that a high level of cartographic quality for PFLP can be achieved through the integrated approach, comparable to the lettering produced by an expert cartographer. The proposed model, expressed as a quality-evaluation function, can be used by any mathematical optimization algorithm to resolve the automated label-placement problem. This article presents a comprehensive multi-criterion model that complies with almost all well-defined cartographic placement principles and requirements for PFLP, allowing for a significant increase in toponym density without affecting legibility. The PFLP problem is still vital, therefore, and solving it is a compelling challenge. As a result, current fully automated solutions are limited in their expressive power. While there have been numerous and varied research efforts to automate point-feature label placement (PFLP), none of them seems to have taken into account the many well-established cartographic precepts for point-feature annotation used by human cartographers.

The lettering process, including assigning names to point features, is an essential part of map production.
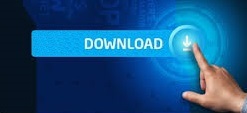